The
Open
Source
AI
Revolution
In the late 20th century, the technology world witnessed a seismic shift as open-source Linux rose to prominence, challenging the dominance of proprietary operating systems from the era’s tech giants. Today, we are on the cusp of a similar revolution in the realm of AI, as open-source language models gain ground on their closed-source counterparts, such as those developed by Google and OpenAI.
In the 1990s, the UNIX ecosystem was dominated by proprietary solutions from major players like Sun Microsystems, IBM, and HP. These companies had developed sophisticated, high-performance systems tailored to the needs of their customers, and they maintained tight control over the source code and licensing. However, Linux, an open-source operating system created by Linus Torvalds, started gaining traction, ultimately disrupting the market.
The Linux revolution was propelled by three key factors: rapid community-driven innovation, cost-effectiveness, and adaptability. By embracing a decentralized development model built off the x86 personal computer, Linux empowered developers worldwide to contribute to its growth. This allowed it to evolve more quickly than its rivals and adapt to a diverse range of applications. Furthermore, Linux’s open-source nature made it significantly more cost-effective than proprietary alternatives, which relied on expensive licensing fees.
Fast-forward to the present day, and we are witnessing a similar upheaval in the AI landscape. The past two months have seen open-source AI projects such as EleutherAI GPT, Stanford Alpaca, Berkeley Koala, and Vicuna GPT, make rapid strides, closing the gap with closed-source solutions from giants like Google and OpenAI. Open-source AI models have become more customizable, more private, and pound-for-pound more capable than their proprietary counterparts. Their adoption has been fueled by the advent of powerful foundation models like Meta’s LLaMA, which was leaked to the public and triggered a wave of innovation.
The Linux saga offers important lessons for the AI community, as the similarities between the rise of Linux and the current open-source AI renaissance are striking. Just as Linux thrived on rapid community-driven innovation built off the backs of the x86 PC, open-source AI benefits from a global pool of developers and researchers who build upon each other’s work in a collaborative manner off the backs of gaming GPUs. This results in a breadth-first exploration of the solution space that far outpaces the capabilities of closed-source organizations.
Another parallel is the cost-effectiveness of open-source AI. Techniques such as low-rank adaptation (LoRA) have made it possible to fine-tune models at a fraction of the cost and time previously required. This has lowered the barrier to entry for AI experimentation, allowing individuals with powerful laptops to participate, driving further innovation.
Moreover, open-source AI models are highly adaptable. The same factors that make them cost-effective also make them easy to iterate upon and customize for specific use cases. This flexibility enables open-source AI to cater to niche markets and stay abreast of the latest developments in the field, much like Linux did with diverse applications.
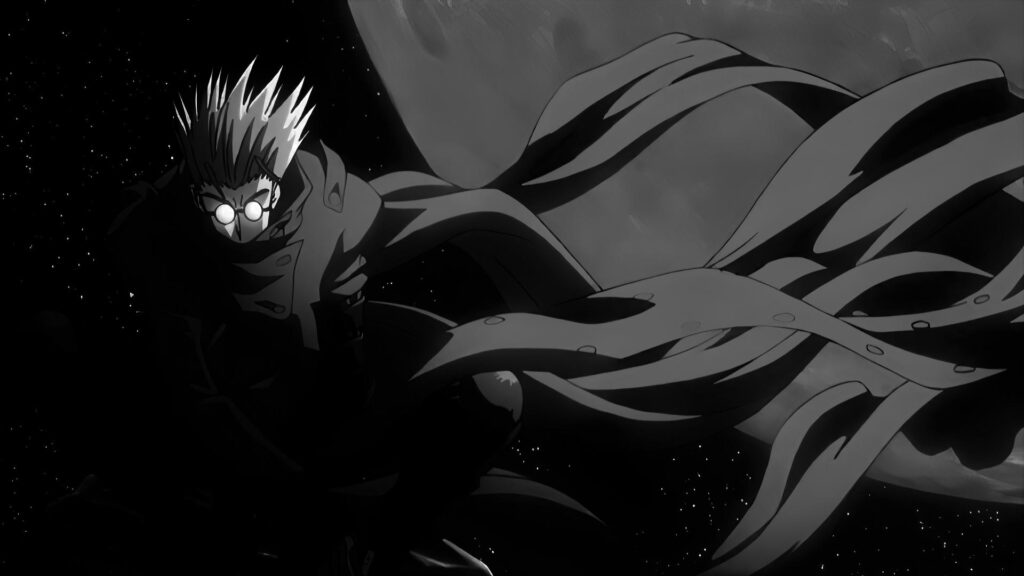
The implications of this open-source AI revolution are profound, especially for closed-source organizations like Google and OpenAI. As the quality gap between proprietary and open-source models continues to shrink, customers will increasingly opt for free, unrestricted alternatives. The experience of proprietary UNIX-based systems in the face of Linux’s rise serves as a stark reminder of the perils of ignoring this trend. In fact, with image generation bots, OpenAI’s Dall-E and Google’s various closed models are barely a point of discussion as the world flocked to open Stable Diffusion models.
To avoid being left behind, closed-source AI organizations must adapt their strategies. Embracing the open-source ecosystem, collaborating with the community, and facilitating third-party integrations are crucial steps. By doing so, these organizations can position themselves as leaders in the AI space, shaping the narrative on cutting-edge ideas and technologies. Companies like Replit, MosaicML, Together.xyz, and Cerebras are doing just that, releasing open-source models, but offering services, finetuning, or operations as a service instead.
“ The implications of this open-source AI revolution are profound, especially for closed-source organizations like Google and OpenAI. As the quality gap between proprietary and open-source models continues to shrink, customers will increasingly opt for free, unrestricted alternatives.”
The flip side of the argument is that this is only possible for a certain model size. There are many emergent behaviors that have only been witnessed on the largest models. While open-source AIs that are an order of magnitude smaller than GPT-3 have already surpassed GPT-3’s quality, this does not necessarily apply to models of the scale of GPT-4 and beyond. With continued scaling in sequence length, parameter count, and training data set sizes, it is possible the gap between open-source and closed-source widens again.
Furthermore, while models are free to use, services that are built on top will still require significant investments. Google, Microsoft, and Meta are able to build these closed-source services for use in people’s everyday lives due to the moat of their platforms. Lastly, the cost of inference is a significant barrier given most consumer devices do not have the horsepower required for models larger than 7 billion parameters (GPT-3 is 175 billion parameters, GPT-4 is over 1 trillion), and it is possible that only the largest organizations can afford to scale their model out to billions of users.
Do you agree with this?
Do you disagree or have a completely different perspective?
We’d love to know