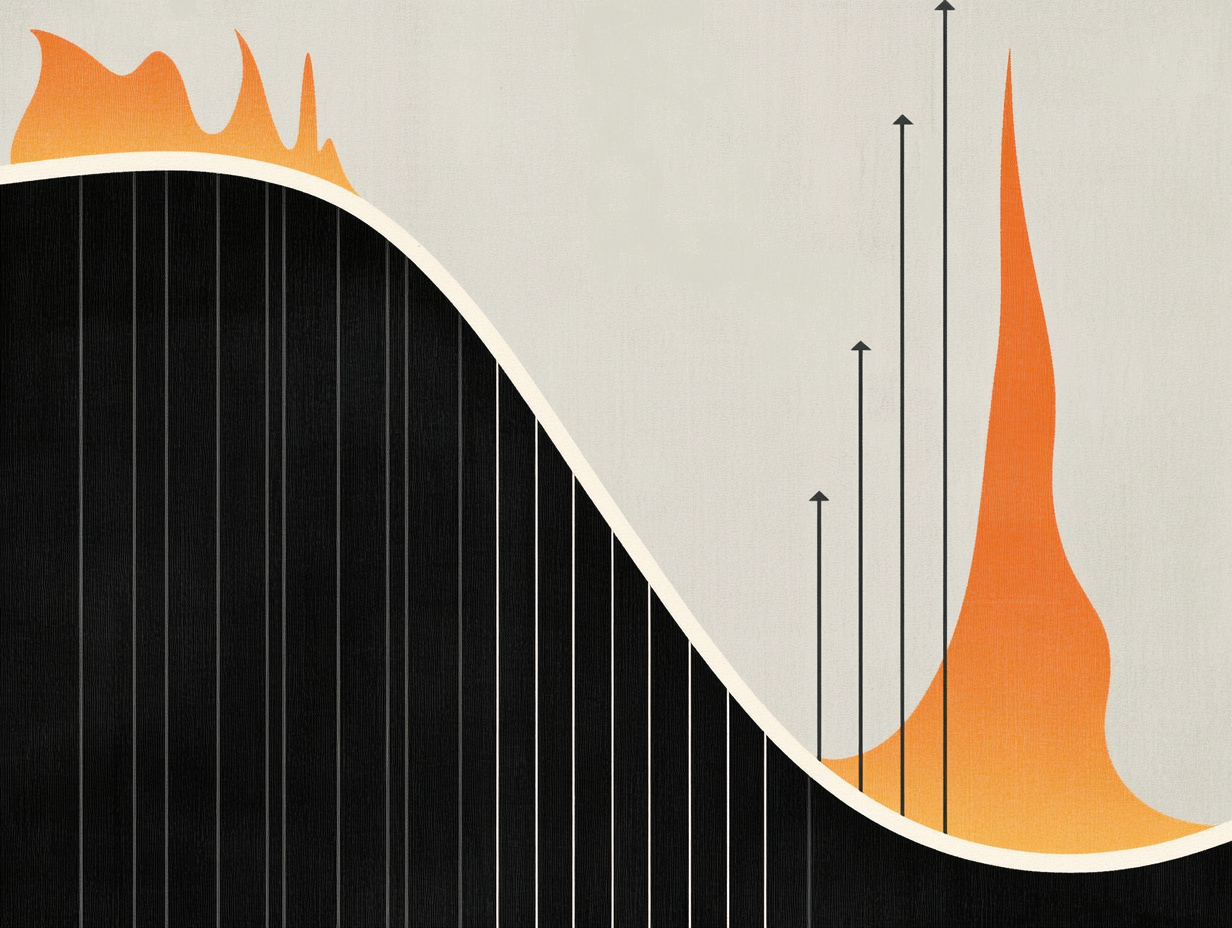
The
Gartner Hype Cycle is Over
We Need a New Paradigm
Editor’s Note: One of the most common references we hear in all of our events is due for a take-down. Our co-founder Toby does his best work when he’s fired up. Check it out and let us know if he is leading you into a 'trough of disillusionment' or a 'plateau of provocations.'
The Gartner Hype Cycle has become the go-to cliché in tech. Not only does this lead to boring, useless perspectives, but it also relies on an outdated research methodology that makes no sense.
To the uninitiated, the Gartner Hype Cycle is a 30-year-old research method that tracks tech adoption over time. Despite its title, the hype cycle is an undulating line (not a cycle) that tracks emerging ideas from the so-called “innovation trigger” through the “peak of inflated expectations” beyond the “trough of disillusionment” and up the “slope of enlightenment” until it reaches the “plateau of productivity.”
If this sounds like the narrative arc of a hero’s journey, that is because the hype cycle is based on fiction. You can read about its origins from its creator here. It was designed to help organizations time and calibrate their investment in developing technology accordingly. The key idea: Early adopters should expect a dip in enthusiasm — the so-called trough of disillusionment — requiring patience and capital that will eventually work its way up into the plateau of productivity.
To be clear, I’m not here to take down Gartner. They successfully leveraged an unprovable narrative arc as a brilliant marketing tool for their services. It’s like a technology horoscope that sounds right 65% of the time. It served its purpose, but now we’re dealing with something bigger.
AI transformation is bigger than hype. It is a super trend. If we plot it on a linear curve, we are obscuring much more interesting considerations.
To navigate AI transformation, leaders need a framework that embraces uncertainty and complexity. I’ve spent the past year interviewing dozens of AI leaders and innovation experts. Their thoughts and observations fit into three states of AI transformation: Possible, Potential and Proven.
Here’s how to envision these three states and the one essential provocation leaders should be asking themselves:
First State:
Things That Are Possible
This is AI’s realm of imagination, where ideas spark but haven’t yet materialized into practical use cases. Theoretical concepts like Artificial General Intelligence (AGI) exist here. Think Hal from 2001. AGI will have human-like cognitive ability.
OpenAI’s focus on AGI reflects the company’s ambition to tackle problems that are decades, if not centuries, away from being solved. And IBM’s neuromorphic computing explorations into brain-inspired chips like TrueNorth aim to mimic human cognition. These chips promise transformative computing capabilities but remain highly experimental. In the First State, research papers and experimental algorithms dominate the landscape, and progress is measured in breakthroughs, not revenue.
If you’re investing here, are you betting on the future or indulging in a fantasy?
Second State:
Things That Have Potential
Here, AI technologies are emerging from academic journals and proof-of-concept stages into early-market experimentation. They’ve demonstrated value but haven’t yet reached a point of reliability or scalability. This is where buzzwords often overshadow results, and visionary leaders must decide how to allocate resources to support fragile ideas.
Tesla’s full self-driving vehicles exemplify this potential. They function in controlled scenarios but remain far from delivering consistent, regulatory-approved results.
Stripe fraud detection with AI, which uses AI to detect and mitigate fraud in online payments, also lives in the Second State. While effective, it requires continuous refinement to adapt to new threats and remain reliable at scale.
Are you willing to nurture fragile, early-stage innovations, or are you only here for the immediate return on investment?
Third State:
Things That Are Proven
This is AI’s gold rush — the domain of scaled, reliable technologies delivering measurable value. Companies here have turned AI from a speculative bet into a fundamental driver of their operations and profits.
Amazon’s AI-driven recommendation algorithms are foundational to the company’s success, influencing customer purchases and optimizing logistics.
Siemens’ predictive maintenance systems in manufacturing ensure operational efficiency, reducing downtime and saving billions annually. And UPS’ AI-optimized logistics, dubbed On-Road Integrated Optimization and Navigation or ORION, optimizes delivery routes in real time, saving millions in fuel and time.
Will you leverage these for the present while building for the future? Or will you let comfort become your cage?
Three Challenges AI Leaders Must Accept
Being an AI leader capable of handling the three states of AI transformation isn’t about frameworks, acronyms or decks.It’s about who you are amid ambiguity, pressure and doubt.
The most productive conversations I’ve had over the past year have involved the three components below. If you drive AI transformation in your enterprise, ask yourself:
1. Can you embrace being wrong?
Leading AI means making calls with incomplete information. You will fail. The question isn’t whether you’ll stumble — it’s whether you’ll learn fast enough to stay in the race. When was the last time you admitted a mistake to your team? If you can’t remember, you’re already in trouble.
2. Are you ready to rethink your identity?
You’re not a decision-maker; you’re a decision-shaper. Your role isn’t to control outcomes — it’s to create environments where great outcomes emerge.How often do you let your team’s experiments challenge your instincts?
3. Can you manage fear — yours and theirs?
AI is a pressure cooker. It amplifies anxieties about jobs, ethics and the future. You can’t outsource courage to a playbook. Leadership means stepping into those conversations, not avoiding them.Have you addressed your team’s fears about AI — or have you assumed their silence means support?
This moment requires breaking out of the regimented ways of thinking. Much like music, classical leadership thrives on precision and control. What’s needed is jazz leadership, which thrives on responsiveness and improvisation. AI’s pace means leaders must constantly adapt to new rhythms and riff on emerging opportunities.
Expecting AI to follow the classical path of the Gartner Hype Cycle will only lead you into a cacophony of failure.
We run events every week. If you want to participate, inquire about membership here. If you want to keep up with the perspectives that we hear, you can subscribe to our weekly newsletter.