The Future is a Simulation
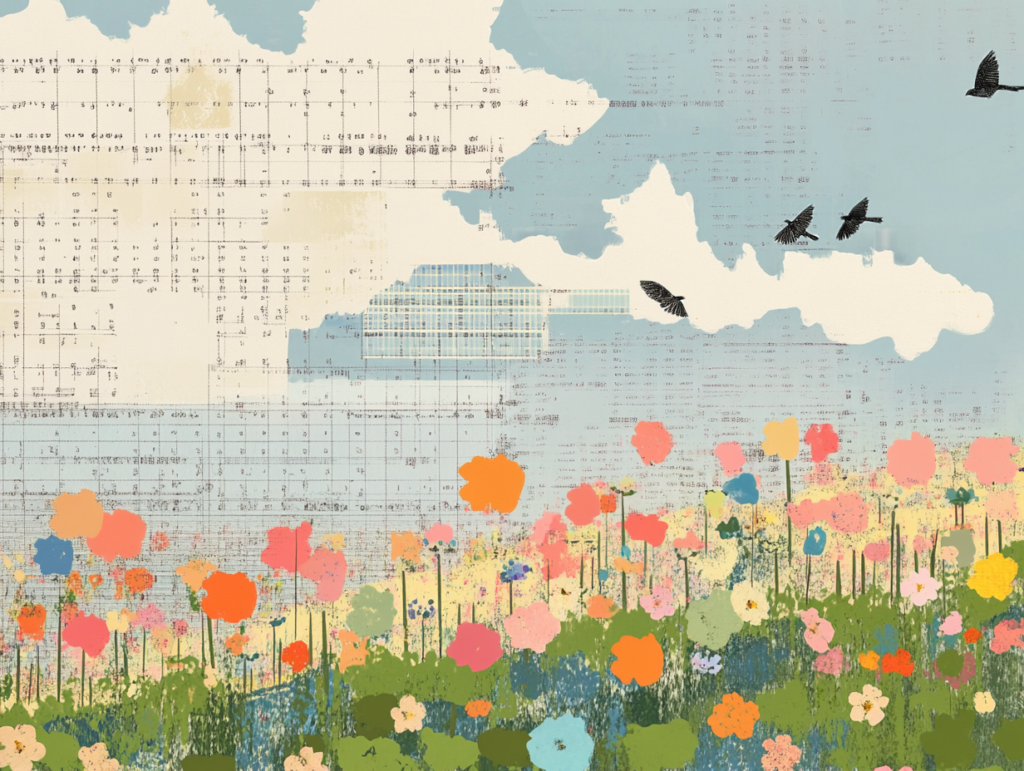
Part 2
The
AI Spring
Editor’s note: The second installment of the Safe to Fail series is a jump-cut from antiquity to the 20th century. The AI spring is mainstreaming simulation techniques that were previously confined to modern-day capital-intensive industries. What can an automotive factory teach a digital platform about simulation?
In the first two decades of the 21st century, before the AI Spring, simulation in the context of innovation remained largely confined to high-risk or capital-intensive industries such as aerospace, automotive, and nuclear energy, where the stakes of failure could be catastrophic both in safety and financial impact. These simulations were resource-intensive, requiring substantial computational power and expertise, which limited their accessibility and application to only those industries that could afford such investments. For instance:
SAFE TO FAIL
The Future is a Simulation
PART 1
An Ancient Strategy
PART 2
The AI Spring
PART 3
Virtual Prototyping
PART 4
Focus Areas and Tools
PART 5
How to Get Started
1. In the pharmaceutical industry...
... computational simulation has broad applications including predicting drug interactions with biological targets, optimizing clinical trial designs, enhancing personalized medicine approaches, and supplementing regulatory approval processes.
2. In the automotive sector...
... simulating the design of new manufacturing lines for electric vehicles can significantly reduce production errors and streamline operations, safeguarding investments that often exceed hundreds of millions of dollars.
3. In the oil industry...
... simulation is used for planning and executing offshore drilling projects by predicting geological conditions and optimizing drilling strategies.
4. In climate science...
... simulations are used to model complex interactions in atmospheric and oceanic systems to predict climate trends and extreme weather. These models synthesize data from diverse sources, including satellite imagery and ground-based weather stations, to simulate processes such as radiative transfer and the hydrological cycle. They provide insights into how climate systems react to various factors, supporting both scientific research and strategy, for example, in the context of international climate negotiations and national policy development.
For endeavors that did not meet the highest thresholds of financial or safety risk, other empirical and analytical risk mitigation strategies were more commonly employed due to cost-benefit considerations. Even for projects undertaken by large corporations in the range of $1M-$10M — which is to say, the vast majority — the financial and resource investments required for sophisticated simulations were too difficult to justify. Additionally, the time and training required to develop and implement simulations can delay project timelines, which may be counterproductive in dynamic and fast-paced environments. Consequently, organizations tended to reserve simulation tools for larger, higher-stakes projects where the potential risks, complexity, and benefits significantly outweighed the costs of simulation technology.
The extent of adoption of simulation in 2024 is equivalent to the adoption of computers in 1970: broadly used in specific industries — but not by most people and not in most industries. But as simulation becomes democratized and barriers to adopting simulation are flattened, there is growing potential to apply simulation to a host of new contexts.
AI algorithms can analyze vast amounts of data more quickly and with greater accuracy than traditional methods. This capability allows for more detailed and comprehensive models, enabling simulations that are not only faster but also offer more precise predictions and insights.
The AI Spring will democratize simulation in several ways:
1. Reducing Cost
AI technologies are driving down the costs of simulations. Advances in machine learning algorithms and more efficient computing architectures are enabling more complex simulations to be run at a fraction of the previous costs. This democratization of technology allows smaller companies and those in less capital-intensive industries to adopt simulation tools.
2. Increasing Accessibility and Ease of Use
AI is also making simulation tools more user-friendly and accessible. AI-powered interfaces and automated systems are reducing the level of expertise required to design and run simulations, opening up the technology to non-specialists and integrating it into regular business operations.
3. Expanding Applications
With these advancements, simulations are now being used in new and diverse contexts. For example, in healthcare, AI-driven simulations are used for predicting patient outcomes, personalizing treatment plans, and training medical staff. In urban planning, they assist in everything from traffic flow analysis to disaster response and environmental impact studies. In retail, simulations help in optimizing supply chain logistics, customer experience, and even in predicting market trends.
By reducing the barriers to entry, the AI Spring is significantly broadening the range of applications for simulation, making it a commonplace tool not just for high-stakes engineering projects but also for everyday business and organizational activities. This expansion is expected to continue as AI technology evolves, further integrating simulation into the fabric of modern industry and society.