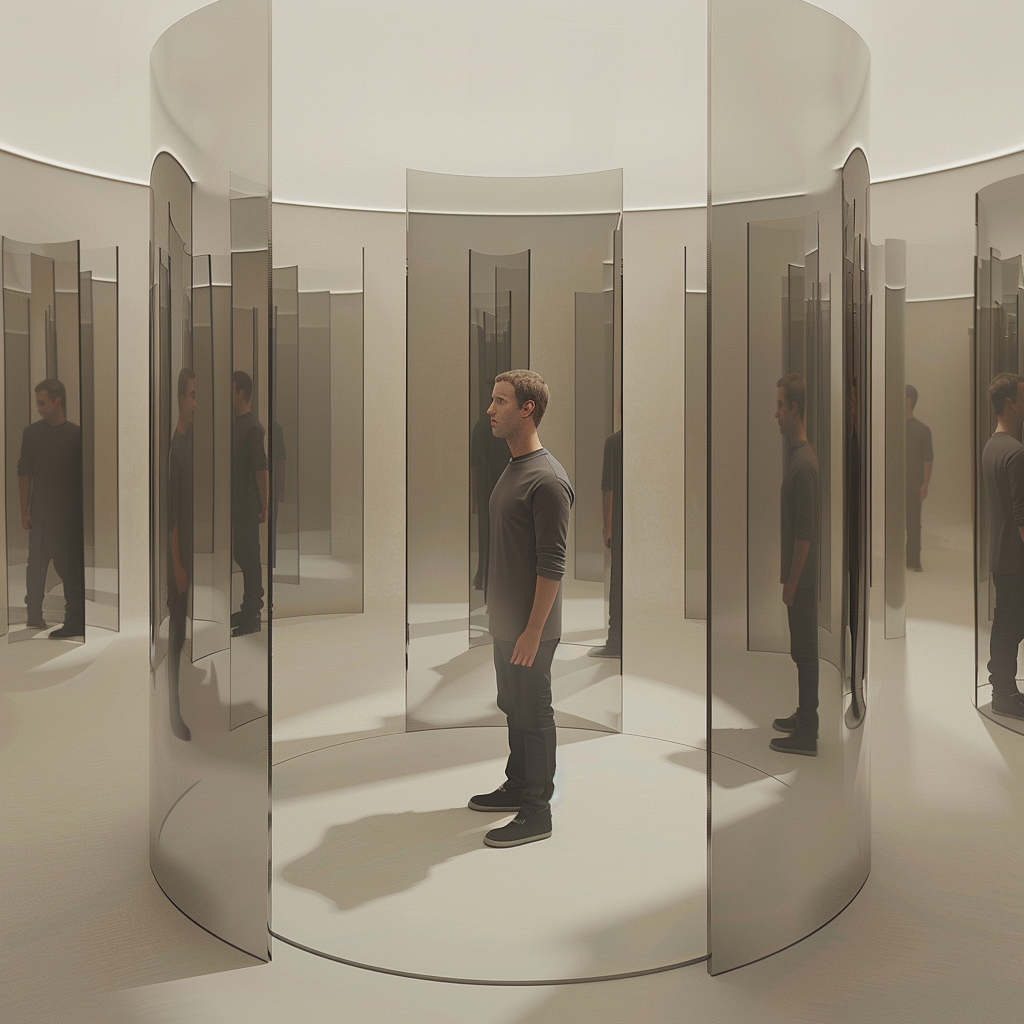
The Infinite
Zuck
How Mark Zuckerberg Became a Shape-Shifter Across Culture—and What That Tells Us About Power Now
Editor’s Note: While everyone was focused on Zuck's coffee habits and his vision for AI companionship, Toby was focused on his code-switching.
Mark Zuckerberg gave three interviews this week. One to Dwarkesh Patel. One to Theo Von. One to Ben Thompson.
Three hosts. Three audiences. Three different cultures of attention.
And somehow, three different versions of the same man.
With Dwarkesh, Zuck was the architect—carefully explaining the inner workings of LLaMA 3, scaling challenges, the logic of open source, and why infra is destiny. A version of Zuckerberg that speaks to the developer class with surgical calm. Less ambition, more constraint. Less metaverse, more compute.
With Theo, he got weird. Not just funny-weird. Existential-weird. He talked about coffee, jiu-jitsu, whether AI can be your friend, and what it means to feel overwhelmed by the world. For a guy who once wore the same grey shirt for a decade, he seemed surprisingly alive here. Vulnerable, even. A human dad, not a techno-overlord.
With Ben, he went back to strategy mode. Threads. Messaging as the new platform layer. Apple’s walled garden. The arc of Meta from feeds to frictionless business tools. This was Zuckerberg as systems analyst, reflecting not just on what Meta is doing, but on what it failed to do. “We just didn’t prioritize the developer ecosystem,” he says, with the tone of someone who won’t make that mistake again.
Same man. Same week. Entirely different presence.
This isn’t a coincidence. It’s choreography.
Power Is No Longer Singular
We used to think of tech founders as having a “core identity.” Jobs had design. Bezos had logistics. Zuck had scale.
But identity doesn’t work that way anymore. In a media landscape where your audience is fragmented, your persona has to fragment too. Zuckerberg is showing us what that looks like in real time. He’s not broadcasting one version of himself to everyone. He’s customizing presence for context.
This isn’t about authenticity. It’s about fluency.
Zuck doesn’t need you to like him. He needs you to recognize him—as credible, legible, and aligned with your values, at least for the duration of the interview. He’ll talk parameter tuning with Dwarkesh, moral complexity with Theo, business model compression with Ben. None of it is fake. But all of it is performative.
He is, in this sense, the first post-founder founder. A man who no longer builds for the internet, but performs on top of it.
So What?
Because this isn’t about Zuckerberg. Not really.
It’s about how power morphs in a world of narrative collapse. When no one voice can dominate, the only ones left standing are those who can slip between voices. Who can code-switch—not just linguistically, but existentially. Zuckerberg isn’t just changing the story. He’s changing who tells it.
The platform once defined the founder. Now the founder becomes the platform.
What’s Next?
He’s done the intellectual web. He’s done Americana surrealism. He’s done strategy’s back room.
So what comes next?
Spirituality? Therapy culture? Gen Z moral philosophy?
Don’t be surprised if you see him on a podcast about neuroplasticity. Or debating Harari on cognition. Or sliding into Twitch streams with creators half his age. Not because he has something to prove—but because he knows that staying still is the surest way to disappear.
That’s the play. Zuckerberg isn’t repositioning the company. He’s reprogramming himself.
He’s testing personas like features. Shipping them like updates. Measuring feedback in trust, not just clicks.
Final Thought
If Musk is trying to be a meme, Zuckerberg is trying to be a mirror.
And maybe that’s the scarier thing.
Because a meme can be ignored. A mirror makes you look back.
And right now, Mark Zuckerberg is reflecting something we might not want to admit: the future belongs to those who can move between worlds without ever claiming one as home.
We run events every week. If you want to participate, inquire about membership here. If you want to keep up with the perspectives that we hear, you can subscribe to our weekly newsletter.