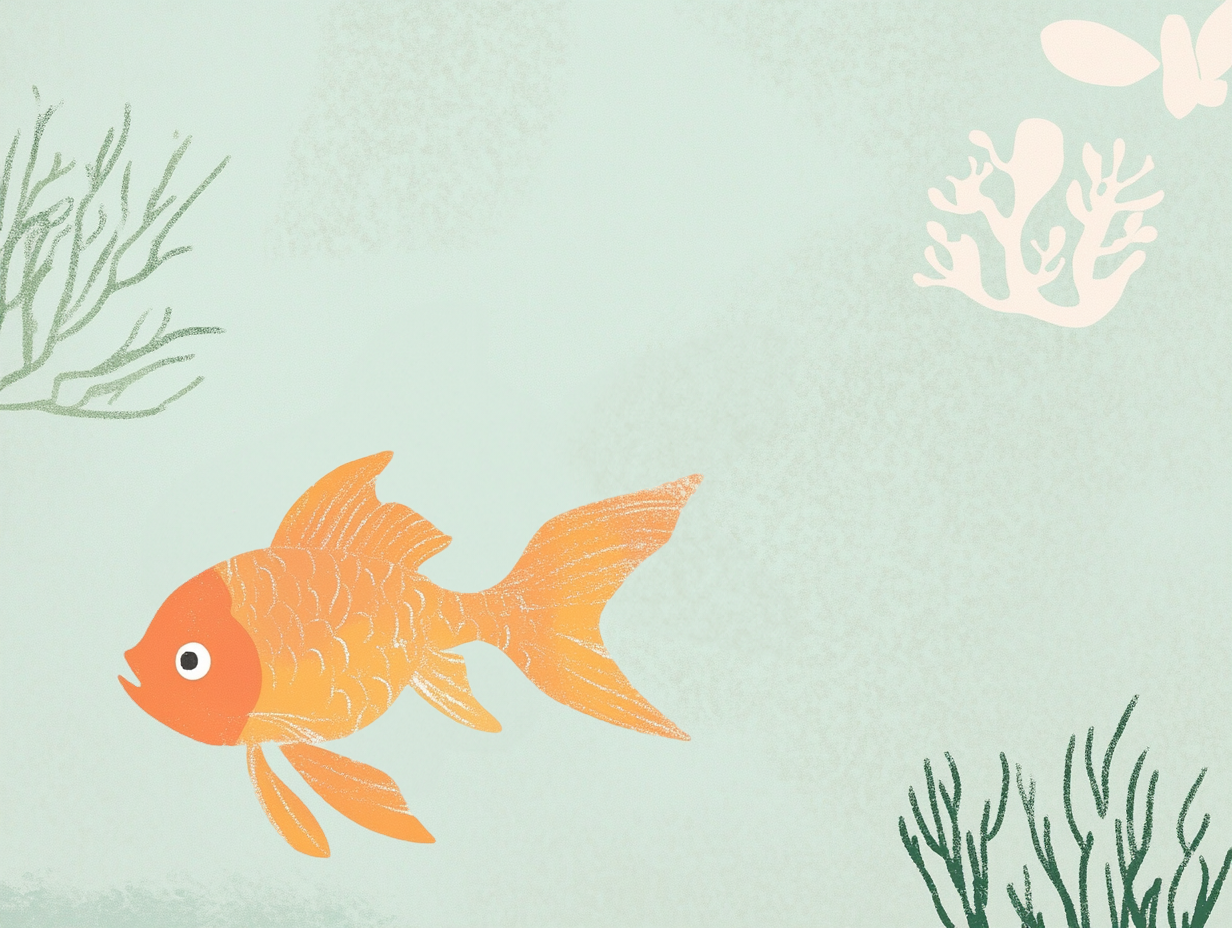
AI Needs
a Feedback
Layer
The next big AI acronym might be: RLHF
Editor’s note: This one caught us by surprise. Sam was in a recent Group Chat dedicated to Gen Z and AI when he suddenly started talking about RLHF. We can’t prove it yet, but Sam might be describing a very important layer to agentic AI that is not yet mainstream.
We are living in an AI gold rush. Instead of shiny rocks, modern-day 49ers are in a desperate search for ‘smart’ features that proactively solve problems and act like a member of the team. And just like a pile of pyrite, these features do not stand up to any kind of scrutiny.
Let me break this down as a scenario.
Your Company Hires a New Agent
On a Monday morning, an employee logs into Slack and is greeted by a message:
"Hi! I’m your new AI teammate. I can help you write client emails, prep briefs, and summarize meetings. Just @ me and tell me what you need."
They type the first prompt:
“Write a quick note to a client thanking them for last week’s meeting and teeing up next steps.”
The reply comes back in seconds. It's… fine. A little stiff. Some awkward phrasing. Definitely not ready to send.
So the employee edits the message. Softens the tone. Adds a reference that the AI missed. Tweaks the subject line. Then sends it off.
A few hours later, they try again. Another prompt. Another almost-right reply. Another round of edits.
This is the invisible labor of AI integration: micro-corrections. Dozens of small decisions made by humans to fix AI.
Is that supposed to be the future?
Are we expecting every employee, in every company, to become an ad hoc AI whisperer - refining, retrying, adjusting - every time they interact with an agent?
Prompting Is a Process, Not a One-Off
Right now, every AI agent is built on the assumption that the prompt is the product. Once it's crafted, it’s deployed. Frozen in time. Maybe a tweak here or there, but mostly untouched, save for the injection of proprietary data.
But real work doesn’t happen like that. Real work requires feedback - constant, messy, iterative feedback.
If you’re a product manager, that feedback becomes feature updates. If you’re a writer, it becomes revisions. If you’re a designer, it becomes pixel-level nudges. But if you’re an AI agent?
There’s nothing. No loop. No memory. No improvement. Just the same prompt, running in place, never learning.
RLHF: The Invisible Architecture of Feedback
This is the blind spot in today’s AI wave. Everyone is obsessed with building smarter models. No one is building smarter systems of feedback — and that’s where the value is hiding.
The thing we learned from decades of digital products — from DTC brands to SaaS platforms — is that conversion is compounding.
- Small improvements add up.
- Friction reveals opportunity.
- Feedback loops outperform static logic.
We need the same logic applied to AI:
- Not just prompt > output.
- But prompt > output > human feedback > refinement > next output.
This is what reinforcement learning from human feedback (RLHF) does at the model level. But applied AI — the stuff showing up in your inbox, your tools, your meetings — has no equivalent.
And that’s the problem.
The Business Layer No One Is Building (yet)
We’re missing a feedback layer in the AI product stack. Not full-blown fine-tuning. Not manual editing forever. We’re missing a lightweight, structured way to capture, score, and re-integrate human feedback.
A system that recognizes:
- Which outputs worked and why
- Which corrections matter most
- How to improve prompts dynamically based on real usage
This is active prompt engineering — a term still under the radar, but rising fast. It treats prompts not as static strings of words, but as evolving systems. Systems that get better over time, just like any good product should.
Why This Matters
The AI gold rush is full of dazzling demos and “smart” features. But when those features get dropped into real companies, the cracks show fast.
Every AI agent is just a frozen prompt until someone teaches it how to learn. And right now, no one is teaching. That’s not a technical oversight. It’s a business opportunity.
The companies that figure out how to design, operationalize, and monetize this feedback layer — they won’t just have better agents.They’ll have learning systems. Systems that adapt. Systems that compound value over time. Systems that feel alive.
This virtuous cycle between humans and AIs is how we will co-evolve together, complementing each other rather than competing.
Final Thought
The AI gold rush is a good thing. It alone is sparking a new infusion of energy and creativity in the digital market. But this rush does not need more pickaxes, it needs more gold pans and sluice boxes and a lot more real-time feedback data. I am not just standing by with a critique; I am building these feedback systems as we speak.
We run events every week. If you want to participate, inquire about membership here. If you want to keep up with the perspectives that we hear, you can subscribe to our weekly newsletter.